The fastMRI dataset—the world’s largest trove of expertly curated, rigorously deidentified, openly shared raw MRI data for machine learning research—now includes a new subset with breast MRI data. The new set and its attributes are described in a paper titled “FastMRI Breast: A Publicly Available Radial k-Space Dataset of Breast Dynamic Contrast-Enhanced MRI,” published in January in the journal Radiology: Artificial Intelligence by a team of researchers from NYU Langone Health; Weill Cornell Medicine; and University Erlangen-Nuremberg.
Although MRI is not the most common type of breast imaging, it is recommended as a screening modality in many countries—including the U.S.—for women who have elevated risk of developing breast cancer.
“With breast MRI, we have this amazing volumetric view of the breast but we also have functional information about the wash-in and wash-out of contrast within the breast,” said Laura Heacock, MD, FSBI, associate professor and director of breast MRI in the Department of Radiology at NYU Langone Health and senior author of the fastMRI breast data.
The technique in question is called dynamic contrast-enhanced (DCE) MRI. As the name suggests, DCE MRI detects the fleeting “enhancement” of tissues as they uptake contrast injected during the exam. The presence, location, intensity, and timing of this enhancement reveal metabolic information not available on mammography, digital breast tomosynthesis, or ultrasound. Cancers, which tap into increasing amounts of blood-borne resources as they grow, light up on DCE MRI because of their voracious contrast uptake. “And that’s why breast MRI has the greatest sensitivity for breast cancer of all the modalities we can image the breast with,” said Dr. Heacock. “That is specifically why we recommend it for women who are at high lifetime risk of breast cancer.”
But DCE MRI could be better: it could be sharper; follow the changes in contrast enhancement more closely; provide a better basis for objective metrics; and take less time. It is advances like these that the authors of the fastMRI breast dataset aim to encourage.
Radial, Dynamic, Ultrafast
The dataset contains deidentified data from 300 clinical DCE MRI exams acquired with a method called GRASP, originally developed at the Center for Advanced Imaging Innovation and Research more than a decade ago with the initial aim of enabling continuous MRI scans in situations that are often complicated by natural body motion. (GRASP has since been incorporated into clinical scanners made by Siemens; become widely used at NYU Langone in neuroimaging and pelvic imaging; and continues to be extended by researchers at NYU Grossman School of Medicine and elsewhere.) GRASP relies on radial sampling, a technique in which MRI signal is acquired in “spokes” that gradually fill k-space—the raw-data dimension from which images are reconstructed—in an asterisk-like pattern. This differs from the common Cartesian sampling in which data are recorded linearly, more like rows filling an array. One of the features of radial sampling is that the spokes, each of which passes through the center of k-space, can be grouped and sorted in various ways after acquisition, providing a tremendous amount of flexibility for the reconstruction of images.
“It’s not the conventional way dynamic contrast scans are acquired today in the clinics,” said Eddy Solomon, PhD, assistant professor in the radiology department at Weill Cornell Medicine and lead author of the fastMRI breast dataset. “You can have, say, 256 spokes during a two-and-a-half-minute scan, and you can decide how many spokes to group consecutively: the first 10, the second 10, and so on—and that will dictate temporal resolution.”
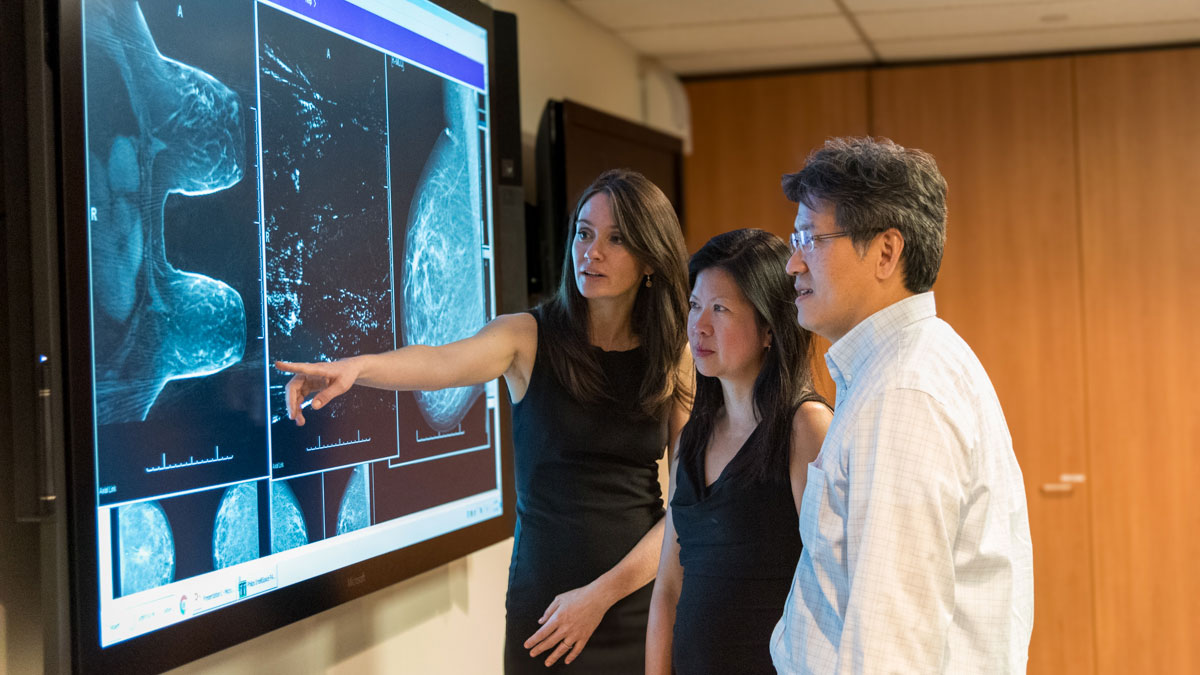
High temporal resolution techniques, known in radiology parlance as ultrafast MRI, produce quick successions of images that give radiologists a moment-by-moment view of contrast dynamics. Speed matters because “some cancers have the contrast so early that by the time we image with traditional methods, they have washed out and are hard to see,” said Dr. Heacock. “The promise of ultrafast is that by taking these rapid-fire images immediately after contrast injection we can catch these cancers and be more suspicious of the findings that are lighting up so brightly in the first minute.” But that promise is up against a fundamental technical tradeoff: “You can either take a beautiful picture or a fast picture”—said Dr. Heacock— “and those are usually not the same thing.”
“When you undersample the data and request such high temporal resolution, you sacrifice image quality,” Dr. Solomon explained. “There are no free lunches.”
GRASP already incorporates several MRI acceleration techniques, such as parallel imaging and compressed sensing, but the researchers are confident that machine learning is poised to further lower the cost exacted by speed on quality. Investigators at the Center for Advanced Imaging Innovation and Research, as well as scientists elsewhere, have demonstrated that deep learning image reconstruction of traditional Cartesian MRI data can accurately generate sharp images from fewer lines of k-space. Scientists at NYU Langone and Meta AI Research have also found that images reconstructed with nearly fourfold acceleration were diagnostically interchangeable with those reconstructed conventionally. The same principles have the potential to advance radial ultrafast DCE MRI, and preliminary research is bearing this out. “We’ve already seen working with this dataset on our own that we can … make the image better using machine learning techniques,” said Dr. Heacock. Now, researchers around the world can use these data in their investigations, too.
Five Treasures
The authors have structured the fastMRI breast dataset to comprise five attributes: raw radially acquired data (and DICOM reconstructed images), clinical case-level labels, high proportion of cases with findings, pre- and post-contrast data, and reconstruction code. Each of these, alone and in combination with others, opens a range of possible lines of inquiry and technical development.
“The unique thing here is that we’re releasing the raw data, and raw data is what gives you the freedom of reconstructing it with almost unlimited flexibility in terms of temporal resolution,” said Dr. Solomon. Raw k-space data is the ground-truth material for image reconstruction, but most publicly available datasets contain only images, not their underlying k-space. Meanwhile, research on image reconstruction conducted in the absence of raw data has been shown to corrupt findings and constitutes what has been coined in the field as “data crimes.” Sharing k-space data not only guards against such pitfalls but also gives scientists the freedom to apply any reconstruction algorithms of interest—“not only GRASP but also model-based, and all the machine learning things.”
Also, “this is the first set that’s radial,” said Dr. Solomon, “which I think speaks more to the MR research and MR physics community.” Radial acquisitions have several benefits, including lower susceptibility to artifacts caused by motion and the already mentioned flexibility, but the reconstruction of radial data demands more computation—hence, time—than that of Cartesian data. Thus, advances in acceleration stand to help bring more radial MRI methods to the clinic.
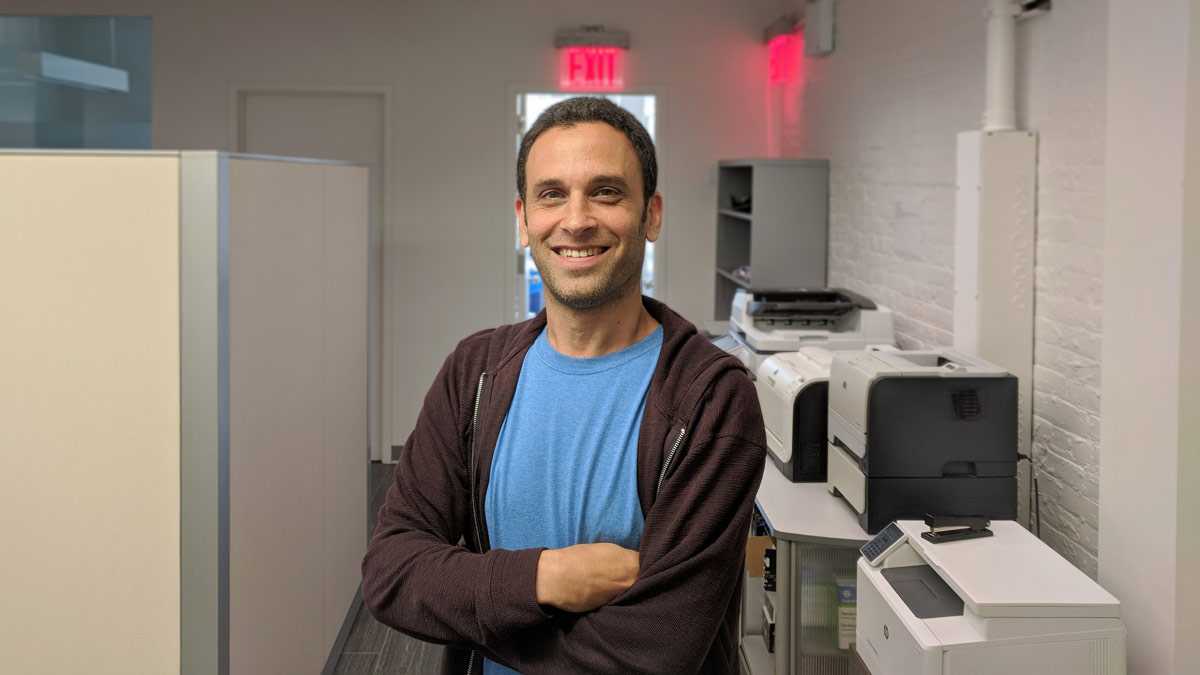
The authors have furnished each exam with rich clinical metadata, including age, menopause status, reason for the MRI, lesion status, and a half-dozen more categories for cases that include malignant findings. Each case has been scrupulously anonymized, so that neither the image information nor the clinical metadata can be linked to any person. The case-level labels make possible research on image classification. Another standout feature of the dataset, Dr. Solomon said, is the high proportion of cases with findings. Most publicly available breast image datasets include relatively few findings, and scarcity of examples poses a significant challenge to the training and generalizability of artificial intelligence models intended to detect and classify lesions. In fastMRI breast data, more than 80 percent of the cases contain findings (roughly 50 percent benign, 30 percent malignant).
In each of the 300 cases, the MRI was acquired continuously for two and a half minutes before and after contrast injection. As a result, for every exam there are two types of data: no-contrast and dynamic contrast-enhanced. “This also opens new possibilities,” said Dr. Solomon, as it gives researchers the choice of advancing the technical aspects of radial reconstruction for either contrast-free MRI or DCE MRI. For those scientists who develop mathematical models of how contrast interacts with tissues, the dataset “also opens new avenues for research in the whole world of quantitative imaging,” said Dr. Solomon, referring to a long-standing area of inquiry aimed at deriving objective, clinically meaningful metrics from DCE MRI through a process called pharmacokinetic analysis.
“And by the way, another advantage to this dataset is we’re providing the code to reconstruct the data,” said Dr. Solomon. The code, available on GitHub, is a GRASP implementation written in Python, a language widely used in machine learning frameworks. “We we’re easing the process for researchers,” he said.
Largest, and Growing
The fastMRI dataset, the world’s largest open collection of expertly curated, meticulously anonymized k-space MRI data, was launched in 2018 by scientists at NYU Langone’s radiology department and its Center for Advanced Imaging Innovation and Research in order to encourage artificial intelligence advances in image reconstruction—part of a collaboration with Meta AI Research to make MRI scans significantly faster.
In short order, the set attracted scientists looking for voluminous, high-quality data with which to develop, train, and test deep learning architectures. According to Scopus, the dataset has so far been cited in more than 260 peer-reviewed articles by authors affiliated with more than 120 institutions in more than 30 countries. Deep learning MRI reconstruction, a relatively fringe area of inquiry in 2018, is now a significant research direction in the field. Several advances made in the course of open competitions and other research enabled by the fastMRI dataset—both at NYU Langone and elsewhere—have since become the foundation of AI-driven reconstructions incorporated into clinical MRI scanners.
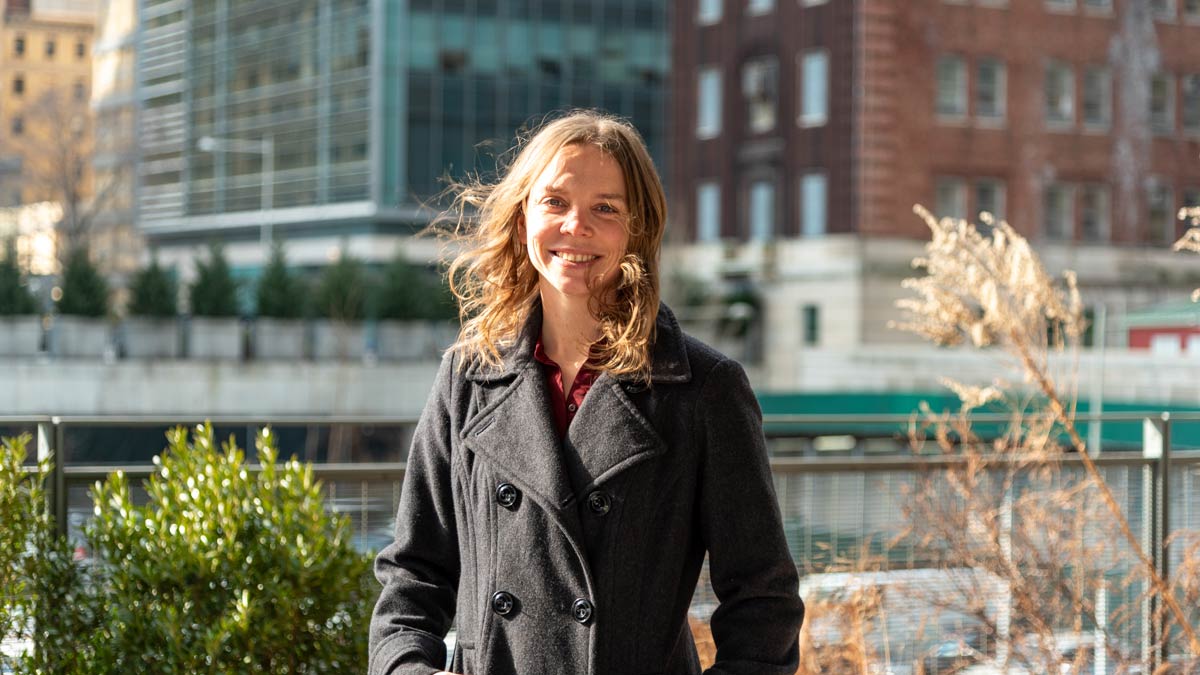
Augmented several times since launch, the collection includes subsets with MRI of the knee, MRI of the brain, diffusion MRI of the prostate, and now also the radial DCE MRI of the breast.
“That really contributes to the diversity of the fastMRI dataset,” said Patricia M. Johnson, PhD, assistant professor in the Department of Radiology at NYU Langone, scientist at the Center for Advanced Imaging Innovation and Research, one of the principal investigators on the fastMRI dataset, and coauthor of the breast data. “It offers new challenges and can open the doors to … techniques that wouldn’t have been possible with the previously published datasets.”
Dr. Johnson expressed the hope that the fastMRI breast data would help researchers come up with creative approaches to improve DCE MRI. “You always have this tradeoff between image quality and temporal resolution, and being able to push the current limits … would be very beneficial. People have done that with fastMRI data before, developing deep learning image reconstruction for better image quality and faster scanning,” she said. “But there’s been less work in that space on dynamic contrast imaging.”
Related Resource
Raw k-space data and DICOM images from thousands of MRI scans of the knee, brain, and prostate, curated for machine learning research on image reconstruction.
Related Stories
Radhika Tibrewala, graduate student in biomedical imaging, talks about the new fastMRI prostate dataset, deep learning in MRI, and how she started a PhD remotely in 2020.
Patricia Johnson, who researches machine learning image reconstruction, talks about faster MRI, visual preferences, and diagnostic interchangeability.
Florian Knoll, incoming chair of imaging at University of Erlangen–Nuremberg, talks about his background, not being greedy, and why he does what he does.