We are sharing MATLAB scripts for L-MPGRE: learned magnetization-prepared gradient echo (MPGRE) sequences used in T2 and T1ρ mapping. This two-level machine learning approach can automatically find parameters of MR pulse sequences to achieve desired performance in key criteria such as accuracy, precision, and signal-to-noise ratio (SNR).
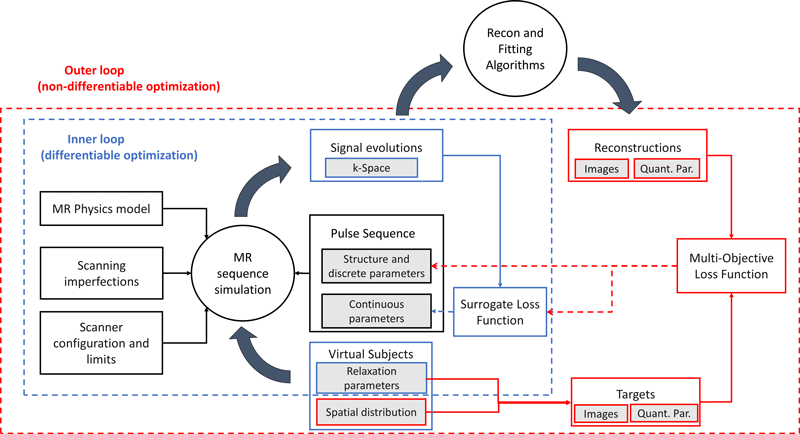
The scripts improve upon our previous methods with two major enhancements:
- Ability to automatically learn discrete and continuous parameters of the MR pulse sequence.
- User options to specify performance goals, such as SNR and quantitative accuracy, through a multi-objective loss function.
In a recently submitted manuscript currently under review, we compare this approach to MR sequences commonly used for mono-exponential, stretched-exponential, and bi-exponential T2 and T1ρ mapping in knee cartilage. One example of such common sequence is magnetization-prepared angle-modulated partitioned k-space spoiled GRE snapshots (MAPSS). In the manuscript, we find that when compared to sequences like MAPSS, our L-MPGRE approach improves the quality of the data while reducing scan time by half.
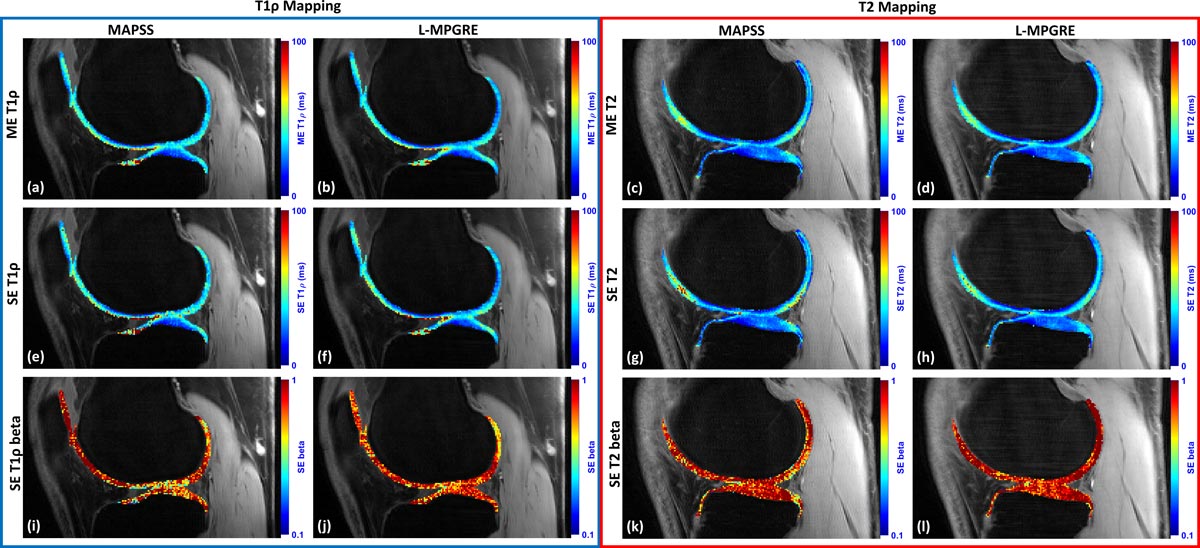
Get the Code
The software available on this page is provided free of charge and comes without any warranty. CAI²R and NYU Grossman School of Medicine do not take any liability for problems or damage of any kind resulting from the use of the files provided. Operation of the software is solely at the user’s own risk. The software developments provided are not medical products and must not be used for making diagnostic decisions.
The software is provided for non-commercial, academic use only. Usage or distribution of the software for commercial purpose is prohibited. All rights belong to the author (Marcelo Wust Zibetti) and NYU Grossman School of Medicine. If you use the software for academic work, please give credit to the author in publications and cite the related publications.
Contact
Questions about this resource may be directed to Marcelo V. Wust Zibetti, PhD.
Related Story
Marcelo Zibetti, imaging scientist at NYU Langone Health, talks about efficiency in MRI, the value of differing vantage points, and learning by thinking across disciplines.
Related Resources
MATLAB scripts for data-driven optimization of magnetization-prepared gradient echo sequences used in T1ρ mapping.
Simultaneous machine learning optimization of parallel MRI sampling pattern and variational-network image reconstruction parameters.
Machine learning optimization of k-space sampling for accelerated MRI.
An accurate, rapidly converging, low-compute algorithm for approximating solutions to the least absolute shrinkage and selection operator (LASSO) problem.