The National Institute of Arthritis and Musculoskeletal and Skin Diseases (NIAMS) has awarded $2.5 million over five years to imaging researchers at NYU School of Medicine for development of deep-learning biomarkers of knee osteoarthritis. If successful, the project has the potential to simplify the search for effective therapies of a disease that affects an estimated 14 million adults in the U.S., where it leads to the majority of over 600,000 knee replacements performed annually.
Osteoarthritis of the knee occurs when cartilage—low-friction tissue that facilitates motion of the joint and protects bones from grinding against each other—breaks down. There is no cure for the disease. Patients are typically prescribed physical therapy, lifestyle changes, and painkillers. At late stages, the only available remedy is total knee replacement.
The search for therapies is complicated by slow, asymptomatic onset of the disease and by its unpredictable, widely variable development.
“We don’t know a priori which patients with early-stage symptoms will progress,” said Cem M. Deniz, PhD, assistant professor of radiology and principal investigator on the project. A decade after diagnosis, some patients retain mobility while others require knee implants. “And we don’t know why,” said Dr. Deniz.
Because of the unpredictable and the chronic nature of knee osteoarthritis, clinical trials need large pools of participants over long periods in order to achieve results statistically significant enough to support conclusions about the effects of experimental therapies. A 2015 analysis of twelve clinical trials found ten of them “underpowered” by hundreds—a few even by thousands—of participants. In addition, methods used in diagnosing osteoarthritis and evaluating its stages often differ among studies. These complexities lead to expensive trials, the results of which are hard to interpret and compare.
Dr. Deniz and a team of co-investigators propose to change that. The scientists plan to train artificial intelligence algorithms to discover objective, measurable biomarkers of early-stage osteoarthritis as well as to predict which patients with early symptoms are likely to experience disease progression within a decade.
The project team comprises experts in machine learning, radiology, rheumatology, biostatistics, and image-analysis software. Co-investigators include: James Babb, PhD; Gregory Chang, MD; Kyunghyun Cho, PhD; Narges Razavian, PhD; Henry Rusinek, PhD; and Jonathan Samuels, MD.
Artificial intelligence methods excel at finding correlations in data but require enormous amounts of information to learn. The project will draw on a cache of data collected by the Osteoarthritis Initiative, a multi-center study aimed at generating resources for further research into knee osteoarthritis. The initiative has amassed millions of X-ray images and magnetic resonance (MR) images, as well as clinical information, such as quality-of-life scores and pain scores, from more than four thousand participants followed over eleven years.
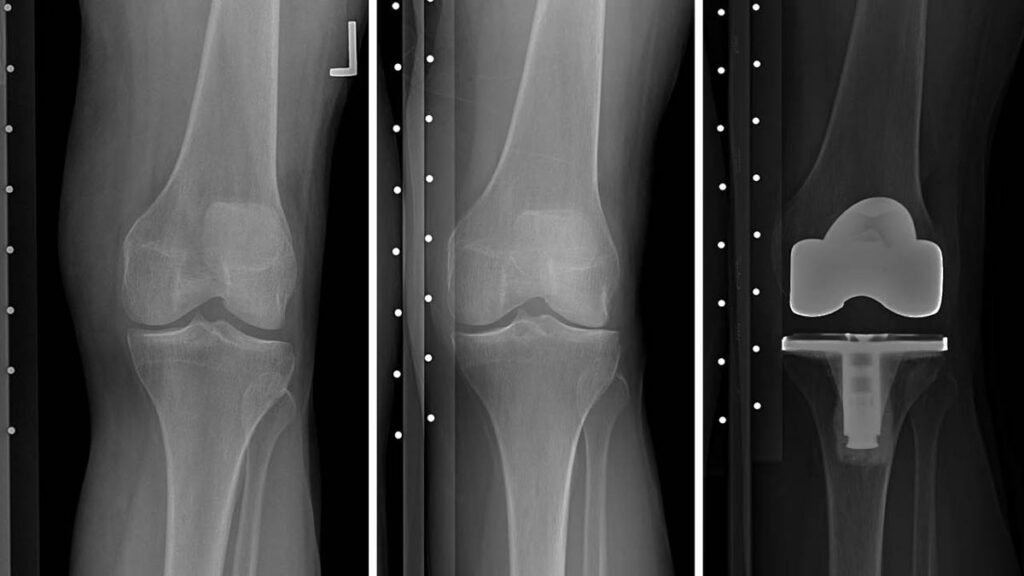
NYU researchers have preselected and annotated information about approximately one thousand participants, matching cases of progression with control cases, and factoring in known correlates of osteoarthritis such as age and sex. This labeled data will be fed into a deep convolutional neural network (CNN) for so-called supervised learning. Unlabeled information from remaining cases will be passed through another part of the CNN for unsupervised learning in which scientists do not direct the algorithm to focus on particular features of the data. The combined approach, known as semi-supervised learning, has been known to produce stronger results than supervised learning alone. The team will also train a recurrent neural network (RNN), which can iteratively store and update its prior states, allowing for comparisons between data from consecutive years.
Clinicians use several means to diagnose osteoarthritis and evaluate its progression. X-ray films can show bone spurs, and, over time, reveal whether the femur and the tibia get closer to each other as the cartilage between them atrophies. Questionnaires allow doctors to assess severity and frequency of patients’ pain. MR images deliver nuanced information about the composition and structure of bones and soft-tissues of the joint. But no one measure has emerged as a reliable indicator of which patients are likely to get worse, and early detection remains difficult.
Research methods for evaluating severity of osteoarthritis exist, but they can be elaborate and time consuming. For example, the MRI Osteoarthritis Knee Score, known as MOAKS, requires a radiologist to examine more than two dozen regions of the knee for bone lesions, cysts, cartilage damage, bone spurs, inflammation of synovial tissue, changes to the meniscus, tendon tears, and irritation of other structures surrounding the joint.
“It takes about 30 minutes per knee,” said Gregory Chang, MD, associate professor of radiology and co-investigator on the project. Imagine the task of comparing such multivariate scores for thousands of knees over the course of a five- or ten-year study.
It is not known how deep-learning networks will affect existing methods, but there are several possibilities. Once the neural network is trained and tested, scientists may analyze it to discover which data are weighed the most. If the network focuses on features that radiologists do not emphasize in their exams, the AI may supplement or change the way clinicians read MR images. If the network can accurately predict progression of disease from one type of MRI, perhaps the full battery of various projections and contrasts will not be needed, potentially resulting in shorter, cheaper imaging studies. Indeed, if accurate predictions can be made on the basis of X-rays and non-image information alone, MRI may become unnecessary, likely reducing research costs even further. But these are hypothetical ancillary benefits.
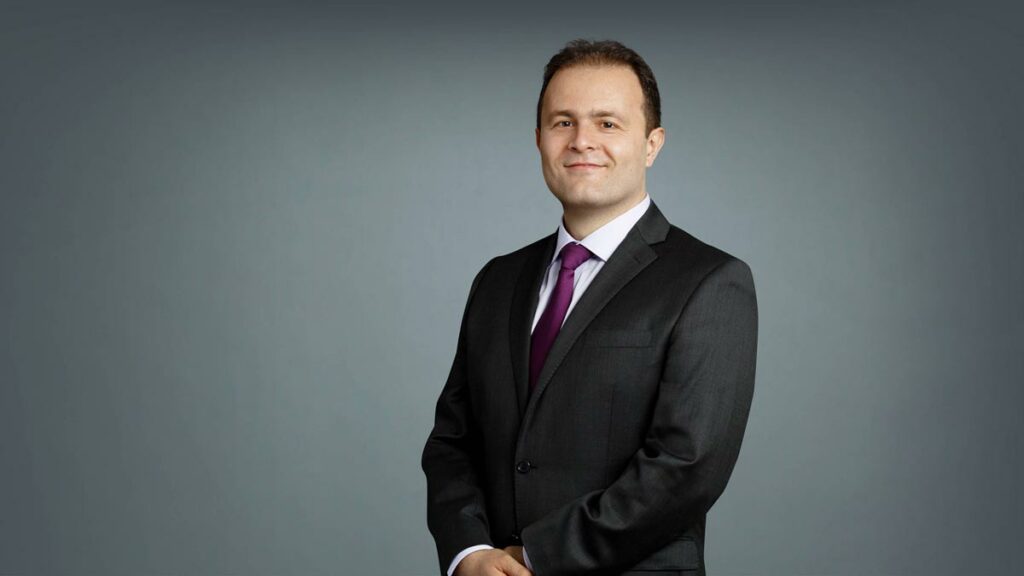
“What I see as the primary outcome of this project is a tool for predicting the OA progression,” said Dr. Deniz, using a common shorthand for osteoarthritis. Such a tool could accelerate clinical trials by taking the guesswork out of participant selection, reducing cohort sizes required for statistical significance, and providing reproducible biomarkers for objective comparison of results. Dr. Deniz envisions an online interface available to anyone interested in research. “By uploading images that you have, you will be able to tell if this person is highly likely to receive a knee replacement or if the OA will progress.”