We are making available MATLAB scripts for estimating diffusion kurtosis tensors and deriving kurtosis parameters from diffusion-weighted images. The toolbox includes (i) a constrained weighted linear least squares algorithm for diffusion kurtosis imaging (DKI) fittings and (ii) introduces a novel DKI estimator for the more robust and reproducible quantification of kurtosis parameters.
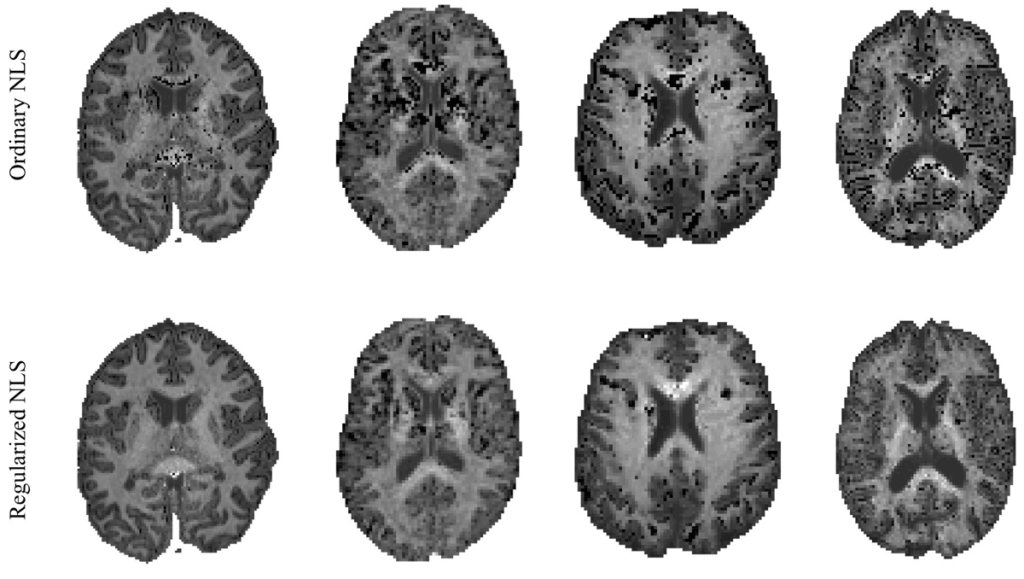
DKI has the potential to become an invaluable tool in diagnostics and clinical research that extends beyond diffusion tensor imaging (DTI) by quantifying the degree of directional non-Gaussian diffusion. DKI parameters have been shown to yield clinically relevant information not captured by more conventional DTI representations. Compared to DTI, DKI requires a slightly more extensive scan protocol in the sense that there must be at least three distinct b-values. Given the widespread availability of accelerated image acquisition techniques, data compatible with DKI-analysis can be acquired in a few minutes, facilitating clinical utility.
Unfortunately, outliers, or notorious “black voxels,” are not uncommon in kurtosis maps. Black voxels result from undesired signal fluctuations caused by motion, Gibbs ringing, or noise, which can often only be reduced using sophisticated tools. Such outliers interfere with visual and statistical inspection of kurtosis parameters maps. If these outliers are noticeable in the resulting mean kurtosis maps, then we suggest the use of the novel robust DKI estimator.
Our novel robust DKI estimator regularizes the tensor fit by using powder-averaged diffusion-weighted data, known as powder kurtosis. This method improves the robustness and reproducibility of the kurtosis metrics and results in parameter maps with enhanced quality and contrast. We hope that these capabilities will promote a wider use of DKI in clinical research and diagnostics.
For best results, we suggest using the DKI estimator after preprocessing the diffusion-weighted data with the DESIGNER pipeline, including denoising and degibbsing, since both steps help to improve the accuracy, precision and robustness of the DKI fitting.
References
Weighted linear least squares estimation of diffusion MRI parameters: strengths, limitations, and pitfalls.
Neuroimage. 2013 Nov 1;81:335-346. doi: 10.1016/j.neuroimage.2013.05.028
Toward more robust and reproducible diffusion kurtosis imaging.
Magn Reson Med. 2021 Apr 8. doi: 10.1002/mrm.28730
Evaluation of the accuracy and precision of the diffusion parameter EStImation with Gibbs and NoisE removal pipeline.
Neuroimage. 2018 Dec;183:532-543. doi: 10.1016/j.neuroimage.2018.07.066
Contact
Questions about this resource may be directed to Jelle Veraart, PhD.